Supervised Machine Learning
FastLending
2021
Project Description
Credit risk is an inherently unbalanced classification problem, as good loans easily outnumber risky loans. Therefore, different techniques were employed to train and evaluate models with unbalanced classes. Imbalanced-learn and scikit-learn libraries were used to build and evaluate models using resampling.
Using the credit card credit dataset from LendingClub, a peer-to-peer lending services company, the first step was to oversample the data using the RandomOverSampler and SMOTE algorithms, and undersample the data using the ClusterCentroids algorithm. Then, used a combinatorial approach of over- and undersampling using the SMOTEENN algorithm. Next, compared two new machine learning models that reduce bias, BalancedRandomForestClassifier and EasyEnsembleClassifier, to predict credit risk.
Related Work
Happy Mutts
Happy Mutts needed an online store designed with full e-commerce capabilities.
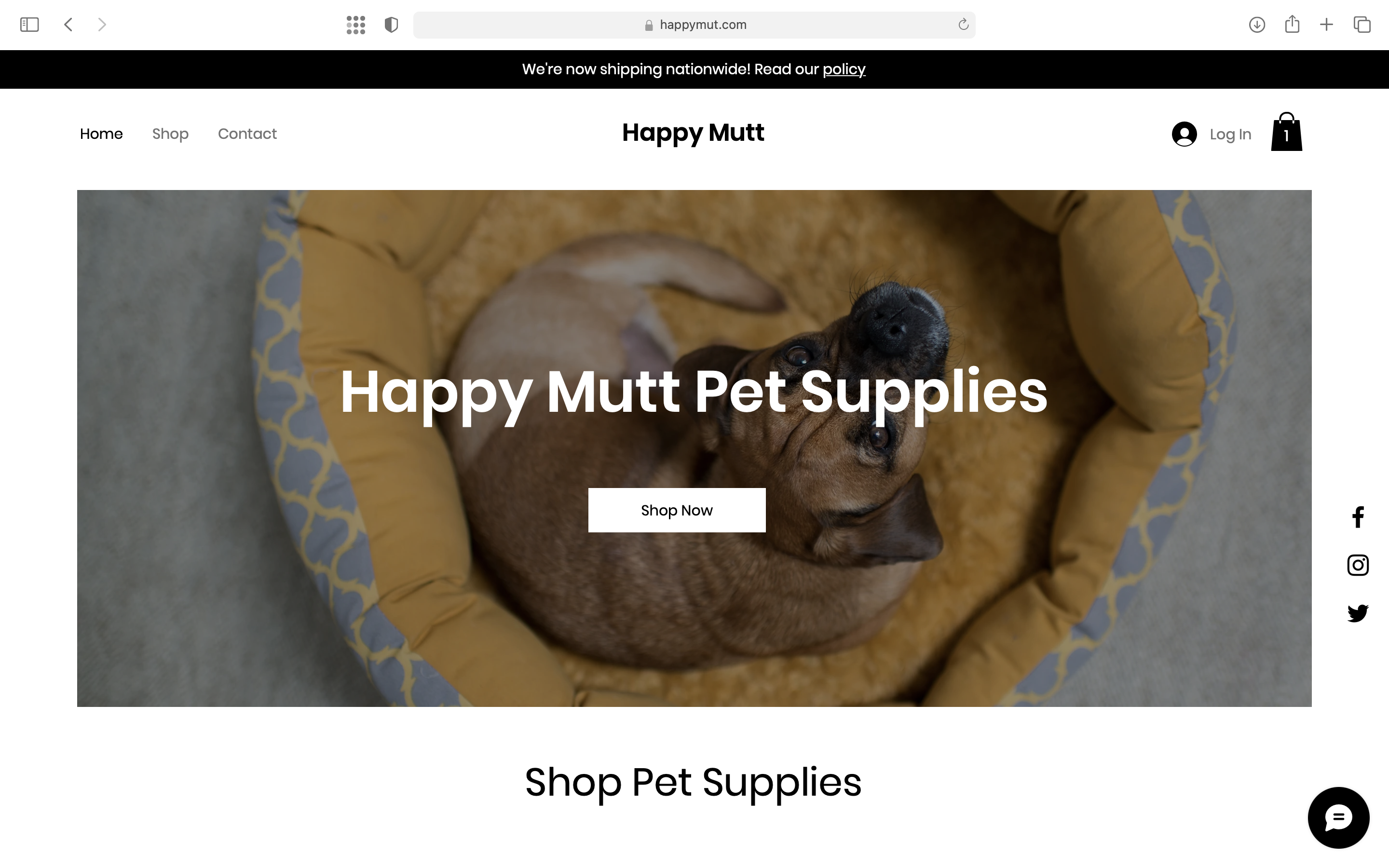
Sunny's
Sunny's Babysitting Services needed a fully functional website designed to provide information to clients and to serve as the main point for booking services.
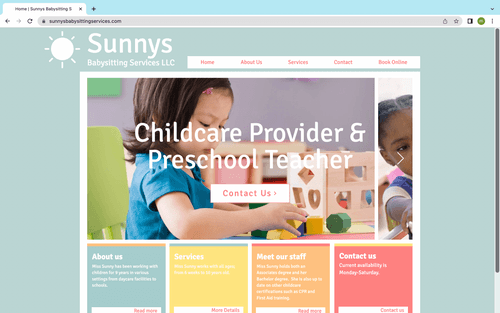
Release and Unleash
Release and Unleash needed an overhaul of their outdated website. The team redesigned and developed a multi-page e-commerce site with a orimary goal of telling the story of Release and Unleash and providing an online store where customers can find their products.

D3 Trucking
D3 Trucking needed a website designed to help with the application process, booking consultations, and selling their digital booklets.

Crypto Analysis
Unsupervised Machine Learning: Clustering
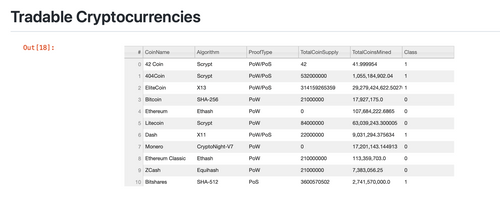

Credit Risk Analysis
The Easy Ensemble AdaBoost Classifier was best at predicting high risk credit applications in comparison to the other models. With a 0.925 accuracy and a 0.91 recall score, both Easy Ensemble AdaBoost and Random Forest Classifiers outperformed the Resampling techniques in accurately predicting high-risk credit card applicants.

Neural Network and Deep Learning
Overall results of the deep learning classification problem was 73% accuracy.
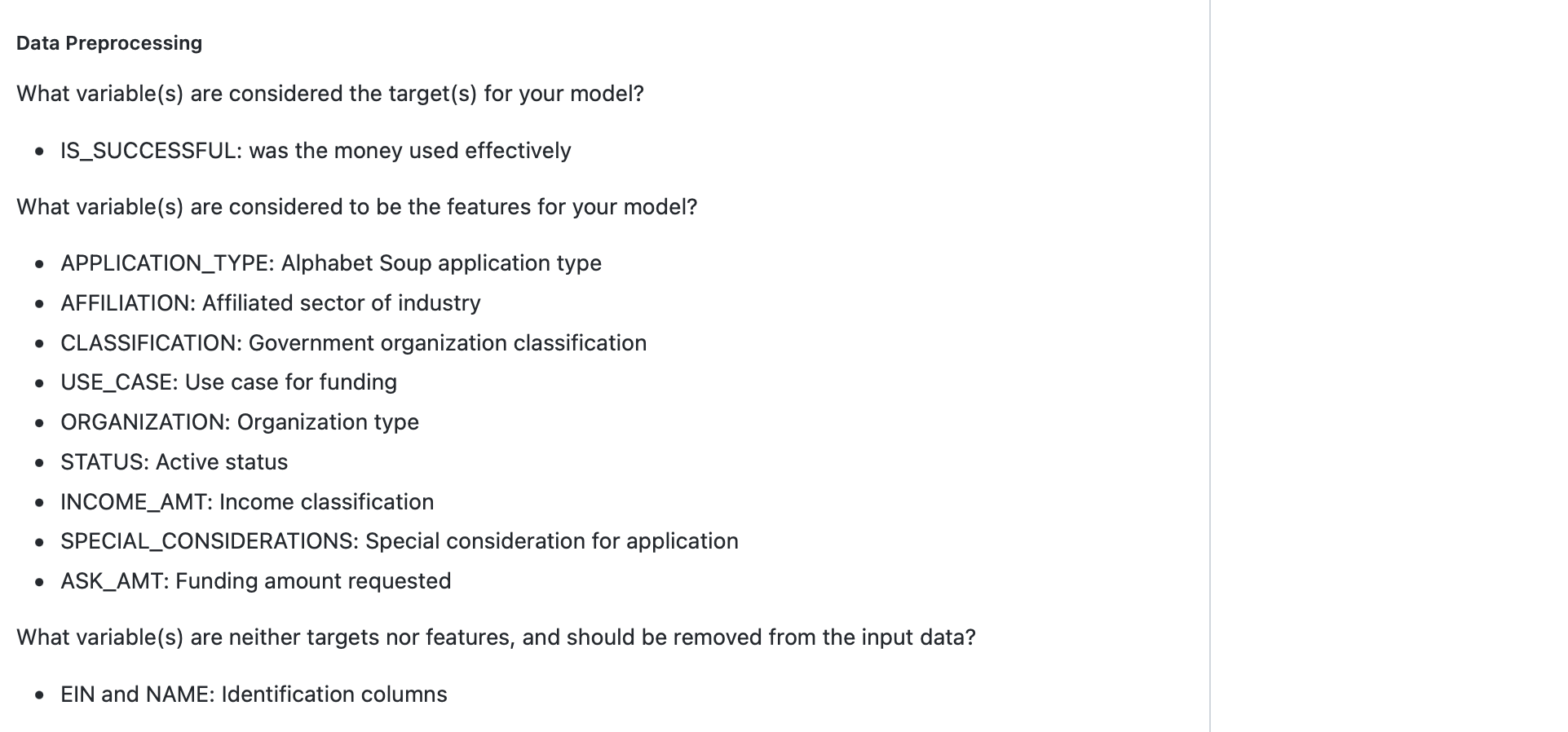